Global Trends in Performance Analysis and Big Data
Advanced use of data is more and more important in the decision making process and It is well researched (Rogers, 2003) that technology adoption tends to follow a curve with 5 major stages (innovators, early adopters, early majority, late majority and laggards). In sport, the rapid investment and adoption of technology means today that almost all Elite organizations use technology to support their performance decision making in some way.
Therefore, because it is no longer an issue of getting access to technology, the next logical step is to think about “what do we do with it that will give us a competitive advantage?”.
This is particularly true in data, where technology today makes it easier than ever to generate and collect data related to athlete and team performance.
These data sources are now rivalling those of other sectors in terms of their depth and breadth - so, those organizations that can make sense of it fastest and apply it within high performance workflows to improve decision making are those that are working within the early stages of the data technology adoption curve.
Providing objectivity at scale
Producing outstanding insights using data that can impact decision making is surprisingly easy BUT only if you have the right people, processes and underpinning technology infrastructure.
In sport, the match calendar is often intense, with matches every few days and the pressure that comes with it due to regular travel and results. It's not uncommon to play 60+ matches each year and this means whatever processes you create to influence decision making must be robust, consistent and scalable to meet these demands. As Aaron Briggs (Assistant Coach at AS Monaco) shared with us:
Case Study: Live Analysis Insights from AS Monaco with Aaron Briggs
With over a decade of experience in football analysis in English Football, including nine years at Manchester City, Aaron Briggs lives every single day in a high-performance environment.
In this article, the now Assistant Coach of AS Monaco details practical insights on how live analysis affects all aspects of the analyst role at an elite level.
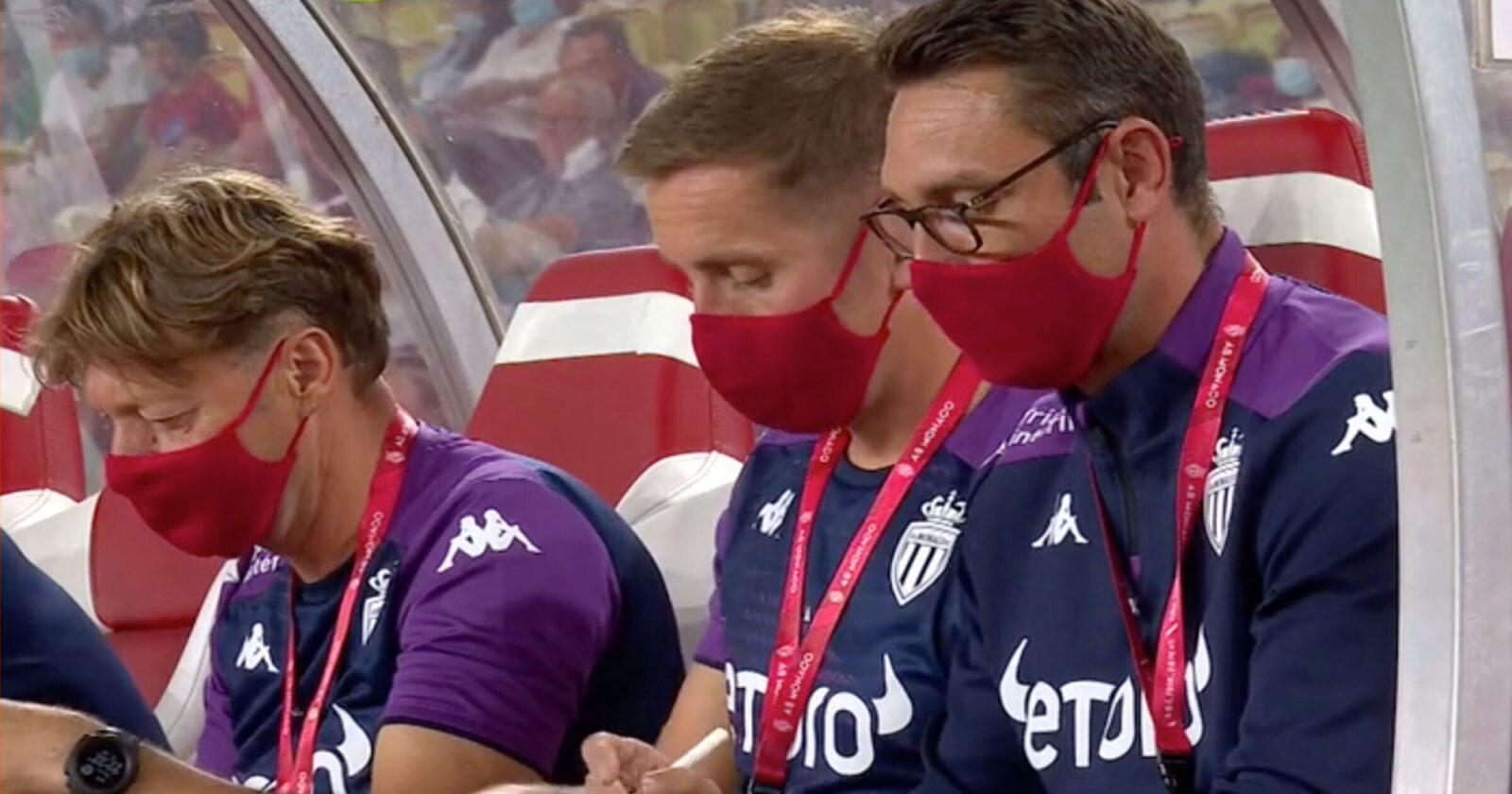
“Consistency across all these different metrics, it's hard work, it's hard work to make sure that everyone's aligned and that everyone understands the process"
Enhancing key high performance workflows
In most organizations, the application of data in high performance workflows starts in player recruitment. Here the challenges are clear, ‘we spend a significant amount of our budget on player decisions, there are hundreds of thousands of players that could be relevant for us, but we can't afford to place a scout in every physical country and league around the world.’
Therefore, data is used to bridge the gaps in player identification and availability of scouts. Done well this means data such as Wyscout API is mixed with other player / team sources into a central database and used to generate outputs that help sports teams take their game model and convert it into a squad planning and recruitment brief process.
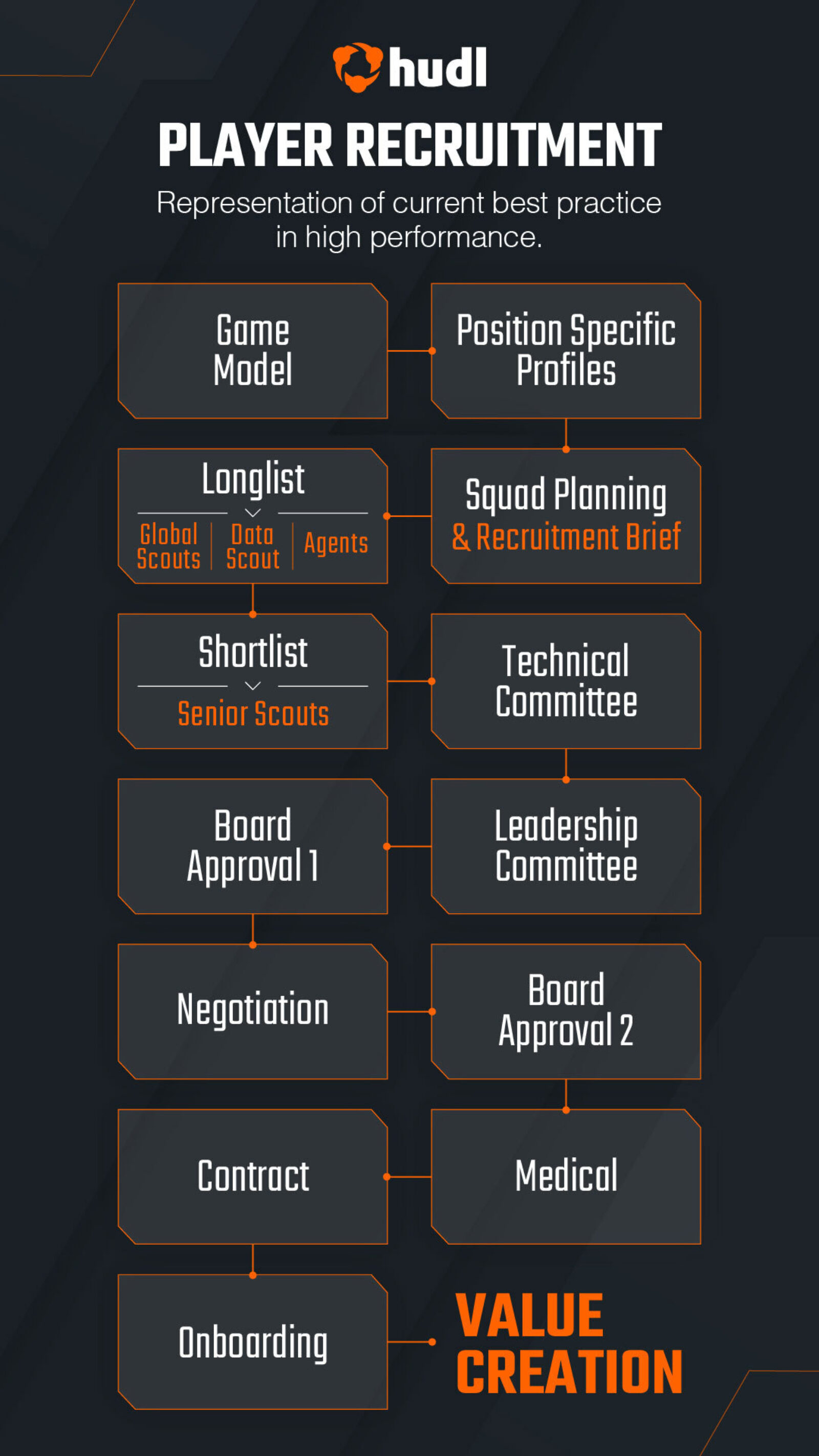
Thereafter, applied data science has now moved into most departments within a high performance sports organization. In coaching, it is helping convert game models and individual player profiles into opposition insights that influence game plans that can be tracked live, and capturing insights related to player individual development plans.
Whereas, in medical and sports science it is assisting rehab and return to training / play protocols by monitoring milestones of physical / mental characteristics vs individual player norms to help maintain clear and healthy relationships with players and coaches.
Increasing efficiency and reducing labour intensive tasks
One of the most common applications of applied data science in sport is identifying labour intensive tasks and automating them. Doing this involves breaking down the task into its stages and then using data science techniques to build models to automate them.
A good example of this is setting up a script to download content from Hudl or Hudl League Exchange at a set time each day so that it's available to be used by coaches, athletes and key support staff. Or using a script applied to Wyscout Video API to automatically download opposition penalties and set pieces so they can then be analysed. OR how Studio in Hudl Sportscode uses Wyscout integration to automatically populate team sheets to speed up telestrations.
Learn more about global trends in modern football in our High Performance Workflows Blog Series